Over the last few years, many organizations have moved toward lakehouse architecture—combining the flexibility of data lakes with the performance of data warehouses. The promise? Unified access, scalable analytics, and AI-ready data.
But for many data leaders, the reality has been disappointing: slow time-to-value, mounting technical debt, and pressure from the business to “show results.” In some cases, data leaders don’t stay in the role long enough to see the fruit of their labour.
So what’s holding the lakehouse back?
ROI gap: lakehouse challenges
Gartner recently outlined the biggest challenges data leaders face when adopting a lakehouse—and these mirror what we hear from enterprise and public sector customers:
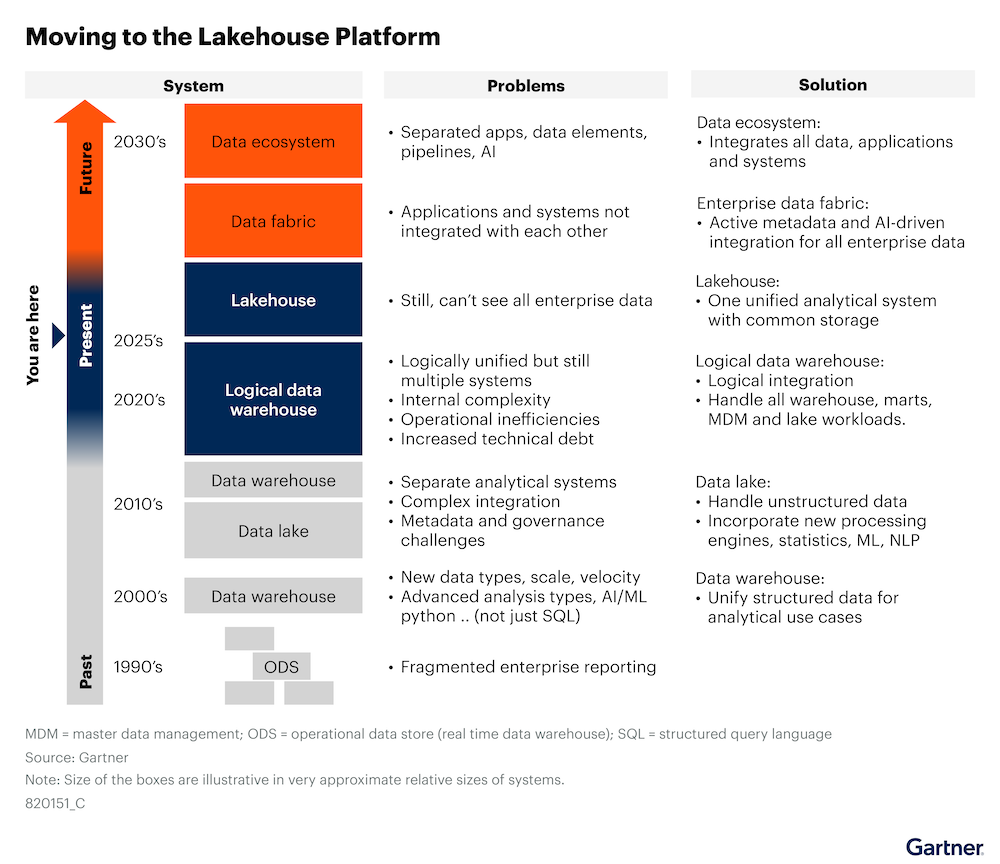
1. Metadata is scattered or missing
A lakehouse is only as good as your ability to find, understand, and trust the data inside it. But many lakehouse implementations lack metadata management. Data remains technical, undocumented, and hard to access, especially for business users.
Example from manufacturing client that spend 60% of IT budget on a lakehouse and…
A manufacturing client had 100+ data sources in their lakehouse, but no searchable catalog or lineage view. Analysts were spending hours just trying to figure out what data existed—let alone how to use it.
2. Data quality is inconsistent or unknown
Lakehouses often inherit raw, unvalidated data from upstream systems. Without built-in data quality checks, errors have no choice but to flow downstream, hurting analytics and AI performance.
Example of a CDO who believed she could run GenAI on top of a lakehouse and …
A retail customer ran a GenAI pilot on their lakehouse, only to find that poor data formatting and duplicates rendered the model outputs unusable. Confidence in the AI initiative led to CDO resigning and not being replaced.
3. No business-facing access layer
Lakehouse adoption often stalls at the technical level. Business users can’t self-serve, and data teams become bottlenecks. Without a semantic layer or natural language access, the lakehouse fails to deliver insights quickly.
A CDO who invested heavily in Snowflake and S3 and …
The only way to access data was through SQL queries. Business teams kept requesting dashboards and real-time analytics that the data team couldn’t deliver although promised at the point of requesting the budget for a datalake, eventually arriving where they started from but had also spent the entire annual budget.
4. Missing data governance and security challenges
4. Missing data governance and security challenges
Lakehouses are complex and still evolving. Many implementations struggle to meet expectations around latency, availability, or fine-grained access control. This creates friction with security and compliance teams and slows deployment.
CDO overpromises and underdelivers….
The same manufacturing client as in example above, had to delay their analytics rollout by 18 months because their lakehouse couldn’t meet internal audit requirements without layering in external governance tools.
Question: can a datalake help answer “Who were my most profitable clients last month and how has this changed compared to 2 months ago?”
Data management practitioners
Answer:
❌ A data lake alone? No, not effectively.
✅ A data lake with proper data management, governance, and analytics layers? Yes.
Stratio: activating value from lakehouse on day one
Stratio helps organizations overcome these blockers by acting as a modular activation layer on top of the lakehouse. Our platform delivers the data management, data governance, and AI-ready capabilities needed to unlock value fast—without disrupting what you’ve already built.
Start with what you need the most right now:
- Need to discover and organize your data, understand what you have and access it in real time?
-> Great, let’s go for giving you tools to collect and manage the metadata and see data clearly in a catalog. - Data quality and lineage missing?
-> No problem, with Stratio data governance module you can monitor, validate, and trust every source. - Business wants to have generative AI capabilities and talk-to-data through questions and answers interface?
-> Excellent, Stratio enables secure natural language querying grounded in all enterprise data with quality, security, and business meaning. - Need to give technical data business meaning to run AI models?
-> Stratio creates a Unified semantic layer for all your data connecting business users to business data.
You don’t need to “go all in.” Stratio is modular by design, meaning you can solve one problem today, then scale into a full platform over time.
From infrastructure to insight and then beyond.
Gartner notes that the “lakehouse is not an endpoint”, but a stepping stone toward the next generation of data architecture: the data fabric.
That’s where Stratio comes in again and proves it delivers the long-term business value too. We don’t just help you activate value from your current architecture—we help lay the foundation for long-term agility, AI-readiness, and innovation across your data ecosystem.
Let’s activate your lakehouse
If you’re sitting on a powerful lakehouse investment but struggling to turn it into business results, you’re not alone.
Stratio can help you:
- Deliver GenAI and analytics use cases in weeks.
- Reduce risk with built-in governance and quality.
- Show value to stakeholders early and often.